Navigating AI Innovation: Why a Proof of Concept Approach Minimises Development Risks
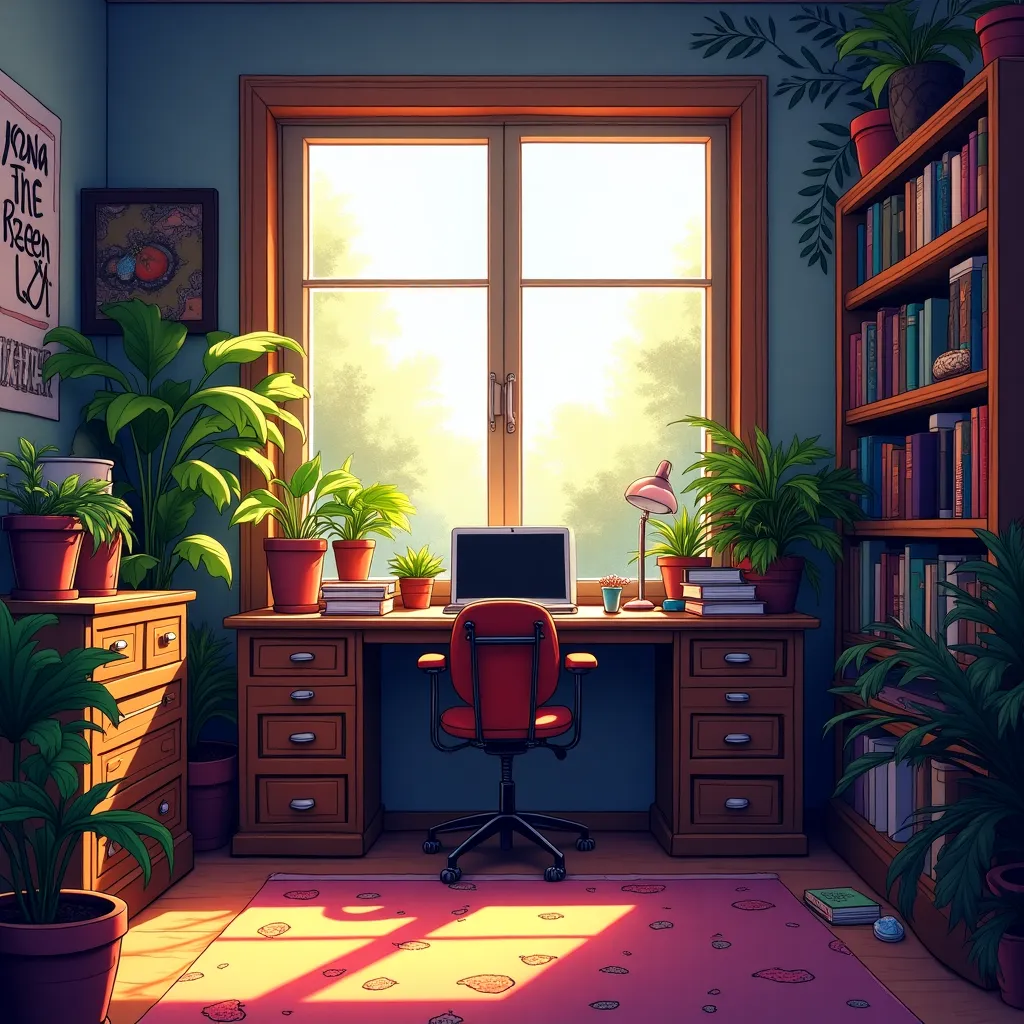
Navigating AI Innovation: Why a Proof of Concept Approach Minimises Development Risks
Embarking on an artificial intelligence journey often presents significant uncertainties. One of our clients managed to preserve three-quarters of their AI implementation budget by beginning with a proof of concept (PoC) approach. The PoC enabled them to validate their concept and identify limitations before committing substantial resources.
When exploring artificial intelligence solutions, always request a proof of concept—particularly if your organisation is new to AI implementation. This strategic approach can save considerable time, resources, and prevent costly missteps.
What Is an AI Proof of Concept?
An AI proof of concept is essentially a small-scale prototype designed to demonstrate the feasibility of a proposed artificial intelligence solution. Its primary purpose is to validate the concept, assess potential benefits, and identify challenges or limitations before full-scale development.
Unlike conventional software proofs of concept, AI PoCs involve more complex considerations, including:
- The system’s capacity to learn and adapt over time
- Potential ethical implications, such as algorithmic bias
- Specific technical requirements for machine learning implementation
When Is an AI Proof of Concept Essential?
An AI proof of concept becomes particularly valuable in these scenarios:
- Your project explores innovative, previously untested ideas at the technical level
- You need to demonstrate feasibility to stakeholders or investors within limited timeframes
- The solution involves complex AI components with uncertain implementation parameters
Key Benefits of the AI PoC Approach
Implementing an AI proof of concept offers several significant advantages:
- Risk mitigation: Identify potential challenges before committing substantial resources
- Iterative learning: Embrace the “fail fast, learn quickly” methodology that enables rapid course correction
- Stakeholder alignment: Generate tangible evidence to secure buy-in from investors and leadership
- Resource optimisation: Uncover process-related issues early, allowing adjustments before full-scale commitment
- Technical validation: Test your proposed technology stack on a smaller scale to confirm its suitability
The Eight-Step AI PoC Framework
Step 1: Problem Identification
Begin by clearly defining which business challenges might benefit from AI intervention. Consider:
- Is the problem specific enough for meaningful evaluation?
- Have you attempted to solve this with conventional technologies?
- Do you have the necessary resources to support this initiative?
- What measurable impact will this have on your business?
- What are the potential risks and limitations?
Step 2: Establishing Business Objectives
Connect potential AI implementations to specific, measurable business objectives. For example, in manufacturing, a measurable objective might be predicting equipment failure with 95% accuracy to reduce maintenance costs.
Step 3: Hypothesis Formulation
Develop multiple hypotheses for potential solutions, which may include:
- Various AI model combinations
- Hybrid approaches that integrate AI with complementary technologies
- Alternative technical architectures
Step 4: Experiment Design
Carefully plan your PoC structure, including:
Data preparation:
- Assess available internal data
- Consider synthetic data generation
- Evaluate open-source or purchased datasets
Environment configuration:
- Select appropriate infrastructure (cloud, on-premises, or hybrid)
- Choose suitable AI development platforms
- Determine testing conditions (simulated vs real-world)
Step 5: Building or Procuring the Solution
Consider whether to:
Build from scratch if:
- Your solution requires innovative approaches
- Existing solutions are prohibitively expensive to customise
- Off-the-shelf options exceed your actual requirements
Procure existing solutions if:
- The total cost of acquisition and customisation is lower than building
- Time-to-market is a critical factor
- The solution closely aligns with your requirements
Step 6: Hypothesis Testing
Conduct rigorous testing through:
- Controlled experiments comparing AI-driven processes against current solutions
- Simulated real-world scenarios that assess practical performance
- Parallel testing of multiple hypotheses when resources permit
Step 7: Value Assessment
Evaluate the PoC against established criteria:
- Measure performance against initial business objectives
- Compare results with pre-implementation metrics
- Benchmark against industry standards
- Gather stakeholder feedback
- Conduct cost-benefit analysis
Step 8: Strategic Decision-Making
Based on your assessment, determine next steps:
- Refine the model and test new hypotheses
- Pivot to alternative approaches if AI proves unsuitable
- Scale the successful PoC to additional business cases
- Proceed to full-scale implementation
Moving Forward with AI Implementation
If your proof of concept demonstrates sufficient value to warrant full-scale deployment, the transition should include:
- Methodical integration of AI models into existing workflows
- Comprehensive testing protocols
- Thorough documentation of architectural decisions
- Continuous monitoring for emerging technologies that might enhance your solution
Why Partner with Kanda Colab for Your AI PoC?
At Kanda Colab, our approach to AI proof of concept development emphasises:
- Time efficiency: We typically deliver initial findings within a fortnight
- Comprehensive documentation: Every decision and outcome is meticulously recorded
- Technology-agnostic recommendations: We prioritise your business needs over specific technologies
- Budget consciousness: We balance cost considerations with performance requirements
- Business-centric focus: Our solutions address your unique challenges rather than showcasing technology
Conclusion
A proof of concept approach allows organisations to navigate the complexities of AI implementation with significantly reduced risk. By testing hypotheses in a controlled environment before substantial investment, you can ensure that your AI initiatives align with business objectives and technical realities.
For organisations taking their first steps into artificial intelligence, a PoC provides invaluable insights that can mean the difference between costly failures and strategic success.